
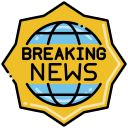
This is a mentally ill person who was driven to an extreme and felt there was nothing better to do than take his own life.
There is no message that should be said other than to urge anyone who is feeling similar distress needs to know that there are people who love them and no matter what there is always a better alternative.
By condoning it for political purposes you give an out for the mentally ill to commit “legitimate” suicide, or worse to being manipulated into doing so. This is not a slippert slope, it is a hard line that many in these comments have crossed - which is why it needs to be said that there is a better path and there are resources.
All images are stacked bar charts if you try hard enough